COMET VS MLFLOW
Finding MLflow Insufficient? Level Up Your MLOps Tooling with Comet!
MLflow is a great tool for teams who are just starting their ML journey. As teams start to deploy models in production, they require a scalable and secure tool like Comet that allows them to reproduce, debug, govern, and monitor their models.
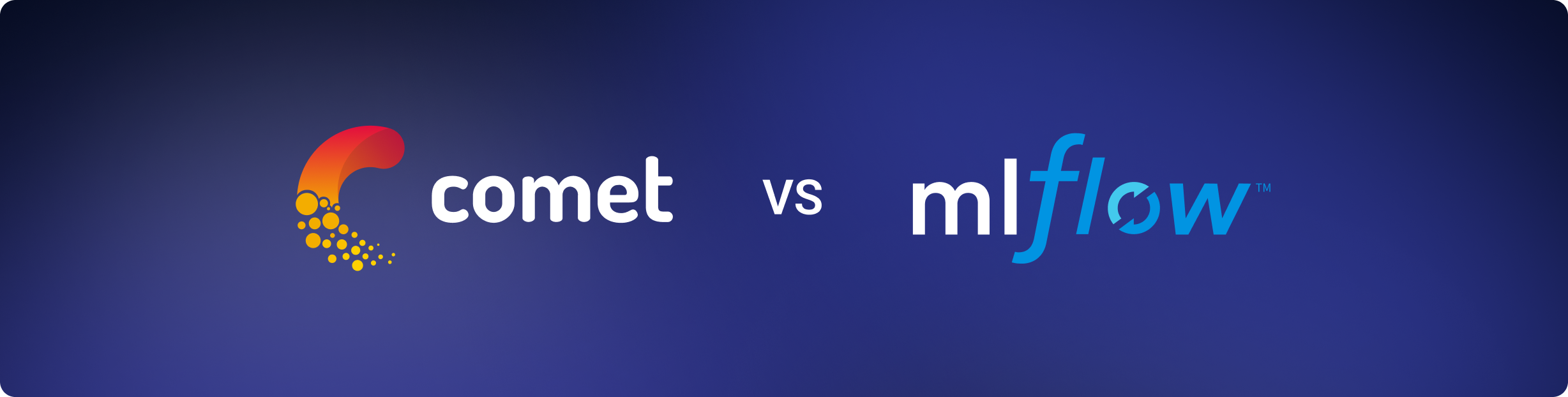
Trusted by the most innovative ML teams
In-Depth Feature Breakdown: Comet vs MLflow
Explore why data scientists and Machine Learning teams are choosing Comet over MLflow.
Feature | ![]() | ![]() | Category |
---|---|---|---|
Deployment Options | Support for VPC/On-Prem Deployments and SaaS hosted solutions | Customer needs to manage backups, scalability and security | Platform |
Scalability | Designed for high scalability: Efficiently handles large datasets and high volume of experiments | Limited scalability, performance issues with high experiment volume, projects with many experiments or big files | Platform |
Collaboration & User Management | Advanced tools for collaboration and admin governance | No notion of user management for teamwork or security | Platform |
Support | Dedicated technical support team | No direct support | Platform |
Compliance Standards | SOC 2 Type 2 compliant and ISO 27001 certified | Documented security vulnerabilities | Platform |
User Interface and Usability | Fast, snappy, intuitive and customizable user interface | Less intuitive UI with usability issues and no customization | Experiment Tracking |
Advanced Visualizations | Hundreds of visualizations available with the ability to build your own | Basic built-in charts | Experiment Tracking |
Integration Ecosystem | Seamless integration with a wide range of popular ML tools and platforms | Limited integrations that requires the user to write a lot of boilerplate code | Experiment Tracking |
Dataset Versioning & Lineage | Robust versioning for model reproducibility and traceability | No dataset versioning | Model Registry |
Model History for Auditing | Documents all necessary information for accountability and transparency in the model lifecycle | No auditing capabilities | Model Registry |
Data Drift Detection | Data drift detection for model input features and output predictions | No production monitoring | Model Monitoring |
Model Fairness Monitoring | Detect model bias across different data segments | No production monitoring | Model Monitoring |
Alerts for Production | Configure customizable alerts for Model Performance degradation in production | No production monitoring | Model Monitoring |
Monitor and manage models, from small teams to massive scale
Frequently Asked Questions
How difficult is it to switch from tracking experiments with MLflow to Comet?
Comet integrates with MLflow! MLflow users will have to change little to none in their training scripts to start logging data to Comet.
Is there a way to retroactively move all the data I have logged in MLflow to Comet?
Yes! We have built migration scripts to make this incredibly easy.
MLflow is open-source and free, why should I pay for a tool?
MLflow has well-known security vulnerabilities, lacks scalability, and has no dedicated support team to help you resolve issues. Fixing all these shortcoming would require a significant resource investment. It’s much easier and cheaper to choose a tool like Comet.