Join us as we explore
THE NEXT FRONTIERS OF
MACHINE LEARNING
At Comet’s Annual Convergence Conference
Virtual Event | May 8-9, 2024
As machine learning evolves, it goes beyond just algorithms generating value; it’s about pioneering uncharted territories of AI. This year’s event dives into the latest breakthroughs and challenges in the field. We will explore the intricate art of building scalable ML platforms, go into the governance of AI, and examine the latest advancements in Large Language Models, Federated ML and Vision Transformers. Our sessions are designed to not only address the technical intricacies but also to explore the broader implications of AI regulation for enterprises and the evolving landscape of AI. This event represents a convergence of thought leaders and innovative data practitioners, all dedicated to charting the course for a more reliable, ethical, and transformative future in machine learning.
Days of content
SPEAKERS
ATTENDEES ONLINE
Join us for this third edition of Comet’s Convergence Conference on May 8-9, 2024 and explore the next frontiers of machine learning, including applications, tools and processes! This two-day virtual event features over 25 expert-led sessions, including in-depth talks, technical panels, and interactive workshops. Engage with leading data scientists and pioneers in the field as we explore the latest developments and discuss AI’s future impact. Be at the forefront of shaping an ethical, transformative ML landscape.
SPEAKERS
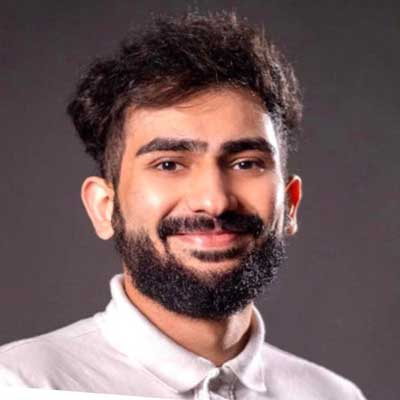
Ameya Diwan
Senior Analyst, Responsible AI, Model Governance at Rocket Central
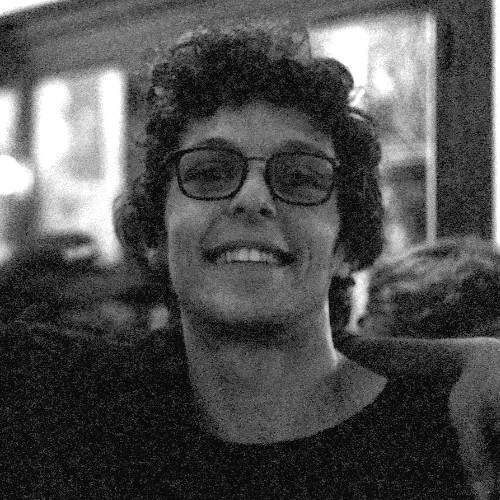
ALEC STASHEVSKY
Lead Scientist, Core Machine Learning at Fetch
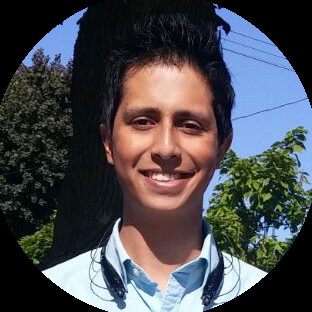
NEIL WADHVANA
Machine Learning Researcher at Torc
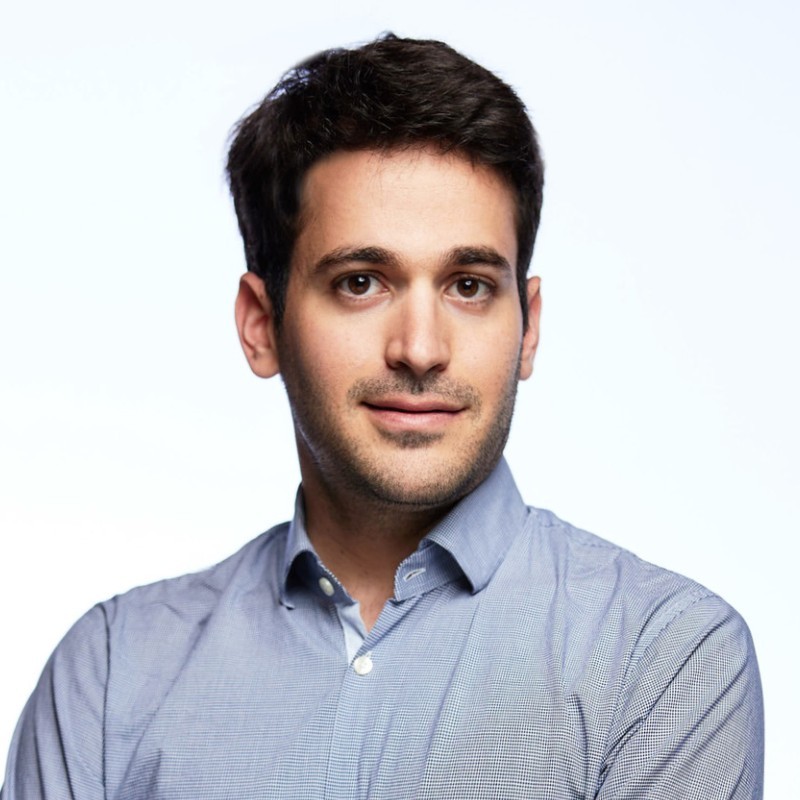
GIDEON MENDELS
Co-founder and CEO at Comet
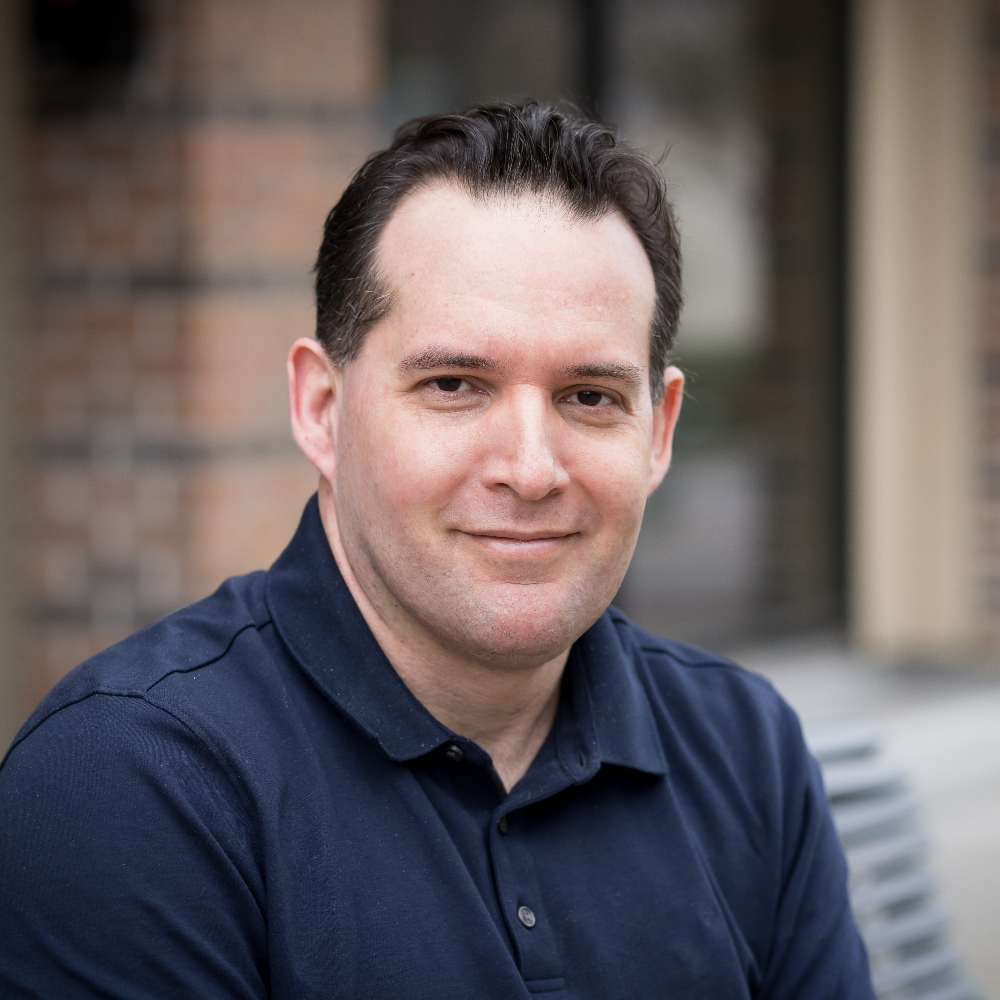
DAVID TALBY
CTO at John Snow Labs
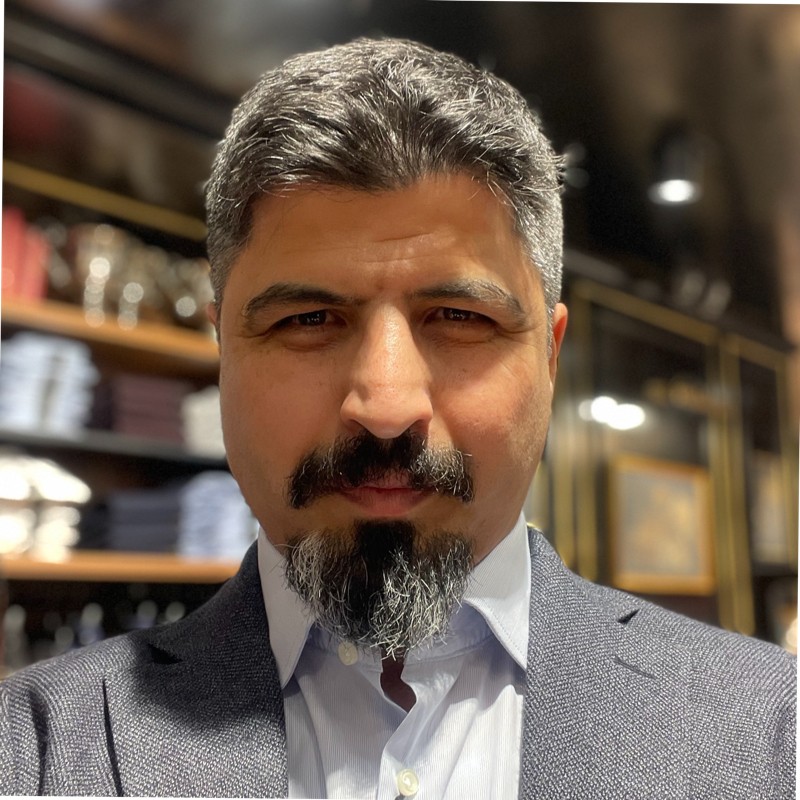
VEYSEL KOCAMAN
Head of Data Science at John Snow Labs
Schedule
Day 1 | Wednesday May 8
12:00PM ET
INTRODUCTION
12:10PM ET
Decoding LLMs: Challenges in Evaluation
Jayeeta Putatunda - Sr. Data Scientist at Fitch Ratings
Large Language Models (LLMs) gave a new life to the domain of natural language processing, revolutionizing various fields from conversational AI to content generation. However, as these models grow in complexity and scale, evaluating their performance presents many challenges. One of the primary challenges in LLM evaluation lies in the absence of standardized benchmarks that comprehensively capture the capabilities of these models across diverse tasks and domains. Secondly, the black-box nature of LLMs poses significant challenges in understanding their decision-making processes and identifying biases. In this talk, we address the fundamental questions such as what constitutes effective evaluation metrics in the context of LLMs, and how these metrics align with real-world applications. As the LLM field is seeing dynamic growth and rapid evolution of new architectures, it also requires continuous evaluation methodologies that adapt to changing contexts. Open source initiatives play a pivotal role in addressing the challenges of LLM evaluation, driving progress, facilitating the development of standardized benchmarks, and enabling researchers to consistently benchmark LLM performance across various tasks and domains. We will also evaluate some of the OS evaluation metrics and walkthrough of code using demo data from Kaggle.
12:40PM ET
Optimizing Sentence Transformers for Entity Resolution at Scale
Alec Stashevsky - Lead Scientist, Core Machine Learning at Fetch
Melanie Riley - Machine Learning Engineer at Fetch
Peter Campbell - Machine Learning Scientist at Fetch
At Fetch, we reward our users for snapping pictures of their receipts. Each day, this happens over 11 million times. Our machine learning and engineering teams are hard at work building systems that extract, normalize, and enrich information from these receipts as accurately and quickly as possible. One of the most important steps in this process is entity resolution. Entity resolution is the process of identifying and linking records that correspond to the same entity across different data sources. Paper receipts have diverse conventions for representing important text entities such as the originating business or "retailer", purchased product descriptions, and payment information. In this talk, we will cover conception to deployment and discuss how we adapt popular sentence transformers and approximate nearest neighbor algorithms to our unique domain of receipt-language. We will discuss how we optimized the models for real-time production workloads and deployed the models to our 18 million MAU. Today the system makes inferences on over 11 million receipts uploaded each day. We also use Comet ML to track our model experiments.
1:10PM ET
Ultralytics YOLO Unleashed: Next-Gen Object Detection in Action
Glenn Jocher - Founder & CEO at Ultralytics
In an era where computer vision is revolutionizing industries, the Ultralytics YOLO (You Only Look Once) model stands at the forefront of this transformation. This session delves into the intricate details of the YOLO object detection system, highlighting its latest advancements and how it seamlessly integrates with various platforms to enable real-time, accurate, and efficient detection capabilities. Attendees will gain insights into practical applications, optimization strategies for different environments, and a glimpse into future developments of the YOLO technology.
1:40PM ET
Developing Conversational AI Agents to Enhance Academic Learning
Sanghamitra Deb - AI & ML Leadership at Chegg
In the past year Generative AI and Large Language Models(LLMs) have disrupted the Education Landscape. We are in the paradigm where AI not only helps with immediate learning needs of students but also plan and design a study guide that can be personalized based on individual needs. Conversational AI agents are perfect for solving this problem. In this presentation I am going to speak about building a conversational learning experience at Chegg. Chegg is a centralized hub where students come to get help with writing, science, math, and other educational needs. In order to impact a student's learning capabilities we are enabling a personalized chat experience powered by an LLM agent. As a user experience this comes in the form of students being able to not just ask questions, but get clarifications on the solution or independent concepts. It’s similar to a tutor helping out with different learning needs. Behind the scenes this experience is powered an LLM agent. An agent is an intelligent system with cognitive abilities to take decisions and make appropriate choices. The first job of the agent is to understand what a student needs when they come to Chegg, they might be wanting quick help when they are stuck on a problem or they could want a study guide for the semester. Once the need of the student is clear the agent has over a decade years of content and student interaction data that it can use and form plans for the conversational learning session. Next the plans are executed by plugging into API such as search and calling traditional Machine Learning models and LLMs from external API and ones fine-tuned on Chegg data. There are several moving parts to building a system that can robustly provide high quality content and scale to millions of students. This requires a robust engineering infrastructure, the agility to adapt to a constantly changing world of LLMs powering the experience and develop a system to monitor and evaluate performance of the conversational AI system. The unique feature of LLMs is they might not give the same result for the same prompt every time, this might cause unexpected behavior when the system is used by millions of students. Building scalable applications with streaming functionality has its own challenges. Fast iterations are extremely important to keep up with the pace of innovation, at the same time creating best practices to ensure accountability and reproducibility is important for experimentation and to create an optimal customer experience. Some of these include prompt versioning, model versioning and monitoring models as they go into production. Another important factor to consider while building an LLM or Generative AI assisted application, does it make sense to build smaller ML models that do classifications, summarizations, NER to reduce the ask from Generative models such that it can scale to larger traffic at lower latency and cost. Is the tradeoff higher development cycles or is it possible to build these models faster using LLM assisted training data? I will address how to answer these questions that come up in the lifecycle of a Generative AI driven application.
2:10PM ET
15 MIN BREAK
2:25PM ET
Panel: Key Trends in AI and Machine Learning to Monitor in 2024
Gideon Mendels - Co-founder and CEO at Comet
Ameya Diwan - Senior AI Ethicist / Senior Analyst, Responsible AI at Rocket Mortgage
Jeremy Ernst - Director, Generative AI Platform Engineering at Ally
Neil Wadhvana - Machine Learning Researcher at Torc
The panel discussion will focus on the latest advancements, ethical considerations, and practical challenges of implementing AI and ML. Attendees will gain a comprehensive overview of the current state and future prospects of AI and ML technologies, alongside strategies for leveraging these innovations for business success in the coming year.
3:10PM ET
Cost Optimizing RAG for Large Scale E-Commerce Conversational Assistants
Anusua Trivedi - Research Director at Flipkart
Mandar Kulkarni - Senior Data Scientist at Flipkart
With the advent of Large Language Models (LLM), conversational assistants have become prevalent in E-commerce use cases. Trained on a large web-scale text corpus with approaches such as instruction tuning and Reinforcement Learning with Human Feedback (RLHF), LLMs have become good at contextual question-answering tasks, i.e. given a relevant text as a context, LLMs can generate answers to questions using that information. Retrieval Augmented Generation (RAG) is one of the key techniques used to build conversational assistants for answering questions on domain data. RAG consists of two components: a retrieval model and an answer generation model based on LLM. The retrieval model fetches context relevant to the user's query. The query and the retrieved context are then inputted to the LLM with the appropriate prompt to generate the answer. For API-based LLMs (e.g., ChatGPT), the cost per call is calculated based on the number of input and output tokens. A large number of tokens passed in a context leads to a higher cost per API call. With a high volume of user queries in e-commerce applications, the cost can become significant. In this work, we first develop a RAG-based approach for building a conversational assistant that answers user's queries about domain-specific data. We train an in-house retrieval model using info Noise Contrastive Estimation (infoNCE) loss. Experimental results show that the in-house model outperforms public pre-trained embedding models w.r.t. retrieval accuracy and Out-of-Domain (OOD) query detection. For every user query, we retrieve top-k documents as context and input them to the ChatGPT to generate the answer. We maintain the previous conversation history to enable the multi-turn conversation. Next, we propose an RL-based approach to optimize the number of tokens passed to ChatGPT. We noticed that for certain patterns/sequences of queries, we can get a good answer from RAG even without fetching the context e.g. for a follow-up query, a context need not be retrieved if it has already been fetched for the previous query. Using this insight, we propose a policy gradient-based approach to optimize the number of LLM tokens and cost. The RL policy model can take two actions, fetching a context or skipping retrieval. A query and policy action-based context are inputted to the ChatGPT to generate the answer. A GPT-4 LLM is then used to rate these answers. Rewards based on the ratings are used to train the policy model for token optimization. Experimental results demonstrated that the policy model provides significant token saving by dynamically fetching the context only when it is required. The policy model resides external to RAG and the proposed approach can be experimented with any existing RAG pipeline. For more details, please refer to our AAAI 2024 workshop paper: https://arxiv.org/abs/2401.06800.
3:40PM ET
Elevating ML Workflows: Harnessing the Power of Our MLOps Platform in an Audience Delivery Company
Jivitesh Poojary - Lead ML Engineer at Comcast
In today's dynamic audience delivery landscape, the seamless integration of machine learning workflows is paramount for success. Join us as we unveil how our MLOps platform revolutionizes audience targeting, optimizing ML workflows to deliver personalized experiences at scale. Discover the transformative power of MLOps in elevating efficiency, accuracy, and agility in our audience delivery endeavors.
4:10PM ET
15 MIN BREAK
4:25PM ET
How to Evaluate a Large Language Model
Debasmita Das - Manager, Data Science at Mastercard
Evaluating Large Language Models presents unique challenges due to their generative nature and lack of ground truth data. Traditional evaluation metrics used for discriminative models are often insufficient for assessing the quality, coherence, diversity, and usefulness of LLM-generated text. In this session, we will discuss several key considerations for evaluating LLMs, including qualitative analysis by human assessors, quantitative metrics such as perplexity and diversity scores, and domain-specific evaluation through downstream tasks. Additionally, we will discuss the importance of benchmark datasets, reproducibility, and the need for standardized evaluation protocols to facilitate fair comparison and advancement in LLM research.
4:55PM ET
Real-Time RAG in LLM Applications
Ankit Virmani - Senior Cloud Data Architect/Field CTO at Google
This talk will go over a real-life example/demo of how to keep the vector DB up to date using streaming pipelines, importance of RAGs and how they can be used to eliminate hallucinations, which can have a huge catastrophic impact on the outputs of LLMs. It would be a great session for data and machine learning engineers would want to learn through a deep dive session on how to fine tune LLMs using open source libraries.
5:25PM ET
WRAP UP
Day 2 | THURSDAY MAY 9
12:00PM ET
INTRODUCTION
12:05PM ET
Generative AI for Healthcare: Hands-on NLP & LLM Training for Data Scientists
Veysel Kocaman - Head of Data Science at John Snow Labs
David Talby - CTO at John Snow Labs
2:10PM ET
15 MIN BREAK
2:25PM ET
DLAI Short Course Workshop: Prompt Engineering for Vision Models
Abigail Morgan - Machine Learning Marketing Engineer at Comet
Caleb Kaiser - Machine Learning Engineer at Comet
Abby is a machine learning marketing engineer at Comet, an MLOps platform for experiment tracking, production model monitoring, data lineage, and prompt management. She also serves as a technical editor and community lead for Comet's online publications. In her spare time, she is a data science and machine learning mentor at Springboard and writes full-code technical tutorials on cutting-edge AI topics. As models grow bigger and more opaque, she is especially interested in observability, explainability, and governance in AI.
4:25PM ET
20 MIN BREAK
4:45PM ET
Workshop: A New Flow in your Workflow: Custom Visualizations Post-Training
Doug Blank - Head of Research at Comet
Dr. Blank is Professor Emeritus at Bryn Mawr College and Head of Research at Comet ML. Doug has 30 years of experience in Deep Learning and Robotics, was one of the founders of the area of Developmental Robotics, and is a contributor to the open source Jupyter Project, a core tool in Data Science.
5:45PM ET
WRAP UP
Some Topics Covered
Federated Machine Learning
AI Governance
Diffusion Models
Scalability and LLMOps
LLM Security
Vector Databases/Search
LLMs in Production
Vision Transformers
Multilingual LLMs
Model Pruning & Distillation
WHY ATTEND CONVERGENCE?
Attending Convergence is the perfect opportunity to be at the forefront of machine learning and AI. This annual conference brings together leading data scientists and industry innovators for a deep dive into the latest advancements, offering expert sessions, interactive workshops, and one of a kind networking. It’s an essential platform for anyone looking to enhance their expertise, stay ahead of emerging trends, and contribute to the ethical evolution of AI.