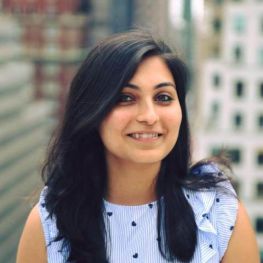
Jayeeta Putatunda
Sr. Data Scientist at Fitch Ratings
Jayeeta is a Senior Data Scientist with several years of industry experience in Natural Language Processing (NLP), Statistical Modeling, Product Analytics, and implementing ML solutions for specialized use cases in B2C as well as B2B domains. Currently, Jayeeta works at Fitch Ratings, a global leader in financial information services. She is an avid NLP researcher and gets to explore a lot of state-of-the-art open-source models to build impactful products and firmly believes that data, of all forms, is the best storyteller. Jayeeta also led multiple NLP workshops in association with Women Who Code, and GitNation among others. Jayeeta has also been invited to speak at the International Conference on Machine Learning (ICML 2022), ODSC East, MLConf EU, WomenTech Global Conference, Data Science Salon, The AI Summit, and Data Summit Connect, to name a few. Jayeeta is also an ambassador for Women in Data Science, at Stanford University, and was a Data Science Mentor at Girl Up, United Nations Foundation, and WomenTech Network where she aims to inspire more women to take up STEM. Jayeeta has been nominated for the WomenTech Global Awards 2020 and has been spotlighted in the List of Top 100 Women Who Break the Bias 2022.
Watch live: May 8, 2024 @ 12:10 – 12:40 pm ET
Decoding LLMs: Challenges in Evaluation
Large Language Models (LLMs) gave a new life to the domain of natural language processing, revolutionizing various fields from conversational AI to content generation. However, as these models grow in complexity and scale, evaluating their performance presents many challenges. One of the primary challenges in LLM evaluation lies in the absence of standardized benchmarks that comprehensively capture the capabilities of these models across diverse tasks and domains. Secondly, the black-box nature of LLMs poses significant challenges in understanding their decision-making processes and identifying biases. In this talk, we address the fundamental questions such as what constitutes effective evaluation metrics in the context of LLMs, and how these metrics align with real-world applications. As the LLM field is seeing dynamic growth and rapid evolution of new architectures, it also requires continuous evaluation methodologies that adapt to changing contexts. Open source initiatives play a pivotal role in addressing the challenges of LLM evaluation, driving progress, facilitating the development of standardized benchmarks, and enabling researchers to consistently benchmark LLM performance across various tasks and domains. We will also evaluate some of the OS evaluation metrics and walkthrough of code using demo data from Kaggle.